Neural Fields and Noise-Induced Patterns in Neurons on Large Disordered Networks
Authors
D. Avitabile, J. MacLaurin
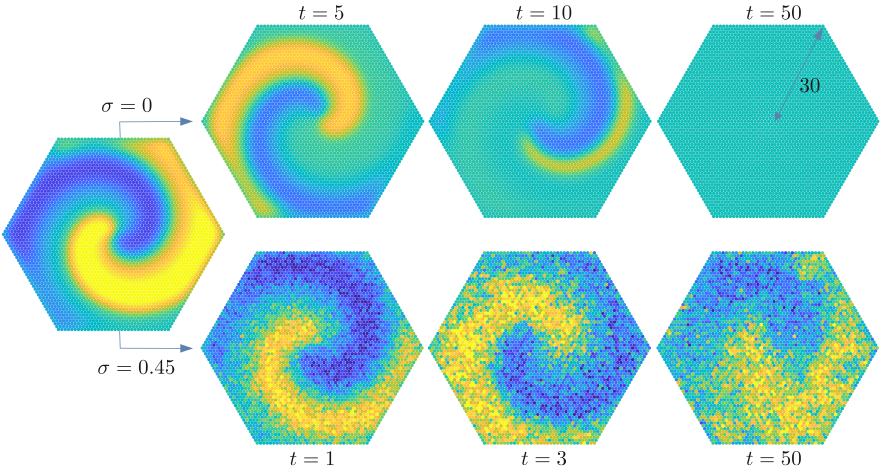
Abstract
We study pattern formation in class of a large-dimensional neural networks posed on random graphs and subject to spatio-temporal stochastic forcing. Under generic conditions on coupling and nodal dynamics, we prove that the network admits a rigorous mean-field limit, resembling a Wilson-Cowan neural field equation. The state variables of the limiting systems are the mean and variance of neuronal activity. We select networks whose mean-field equations are tractable and we perform a bifurcation analysis using as control parameter the diffusivity strength of the afferent white noise on each neuron. We find conditions for Turing-like bifurcations in a system where the cortex is modelled as a ring, and we produce numerical evidence of noise-induced spiral waves in models with a two-dimensional cortex. We provide numerical evidence that solutions of the finite-size network converge weakly to solutions of the mean-field model. Finally, we prove a Large Deviation Principle, which provides a means of assessing the likelihood of deviations from the mean-field equations induced by finite-size effects.
Links
ArxivBibTeX
@misc{avitabile2024neuralfieldsnoiseinducedpatterns,
title={Neural Fields and Noise-Induced Patterns in Neurons on Large Disordered Networks},
author={Daniele Avitabile and James MacLaurin},
year={2024},
eprint={2408.12540},
archivePrefix={arXiv},
primaryClass={math.PR},
url={https://arxiv.org/abs/2408.12540},
}