Next-generation neural field model: The evolution of synchrony within patterns and waves
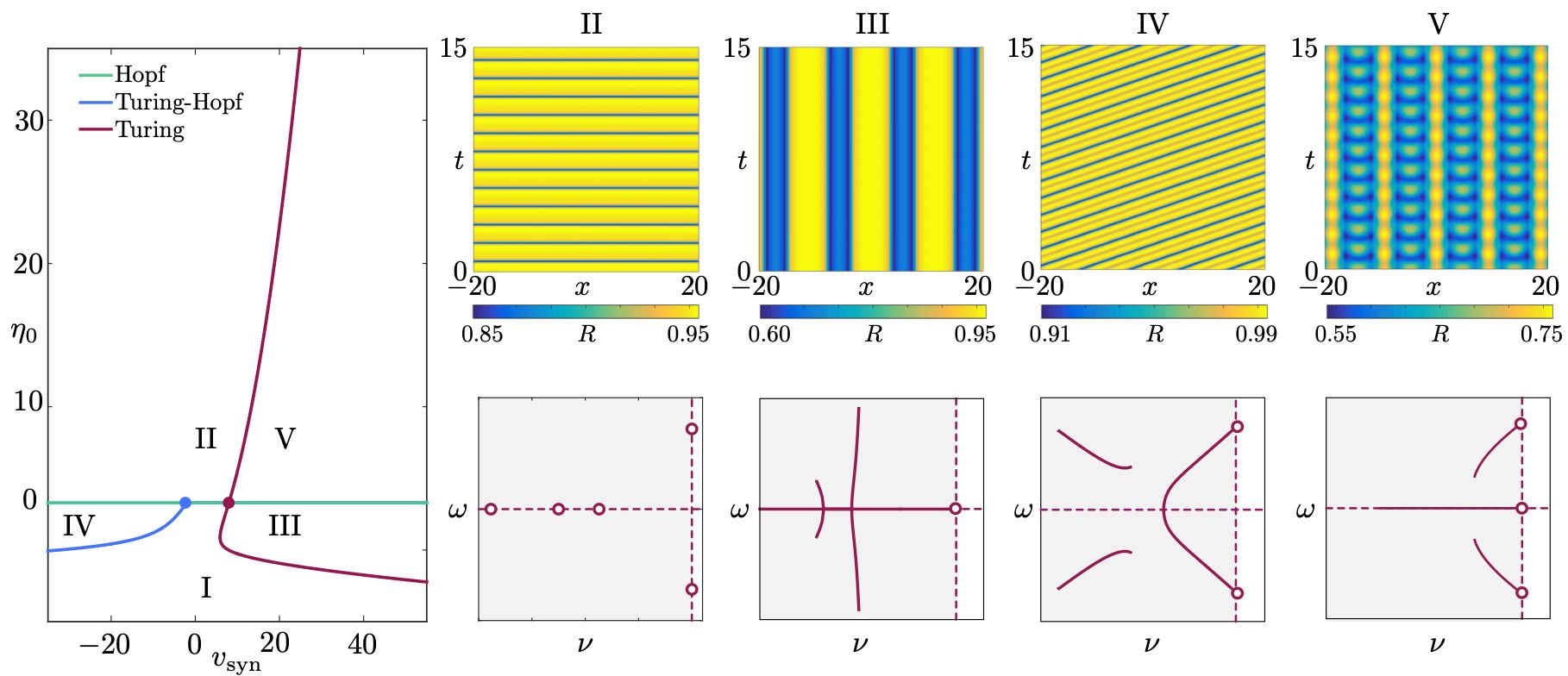
Authors
Byrne, Á., Avitabile, D. and Coombes, S.
Abstract
Neural field models are commonly used to describe wave propagation and bump attractors at a tissue level in the brain. Although motivated by biology, these models are phenomenological in nature. They are built on the assumption that the neural tissue operates in a near synchronous regime, and hence, cannot account for changes in the underlying synchrony of patterns. It is customary to use spiking neural network models when examining within population synchronization. Unfortunately, these high-dimensional models are notoriously hard to obtain insight from. In this paper, we consider a network of θ-neurons, which has recently been shown to admit an exact mean-field description in the absence of a spatial component. We show that the inclusion of space and a realistic synapse model leads to a reduced model that has many of the features of a standard neural field model coupled to a further dynamical equation that describes the evolution of network synchrony. Both Turing instability analysis and numerical continuation software are used to explore the existence and stability of spatiotemporal patterns in the system. In particular, we show that this new model can support states above and beyond those seen in a standard neural field model. These states are typified by structures within bumps and waves showing the dynamic evolution of population synchrony.
Links
DOI PDFBibTeX
@article{byrne2019next,
title={Next-generation neural field model: The evolution of synchrony within patterns and waves},
author={Byrne, {\'A}ine and Avitabile, Daniele and Coombes, Stephen},
journal={Physical Review E},
volume={99},
number={1},
pages={012313},
year={2019},
publisher={APS}
}