Robust working memory in a two-dimensional continuous attractor network
Authors
Wojtak, W., Coombes, S., Avitabile, D., Bicho, E., Erlhagen, W.
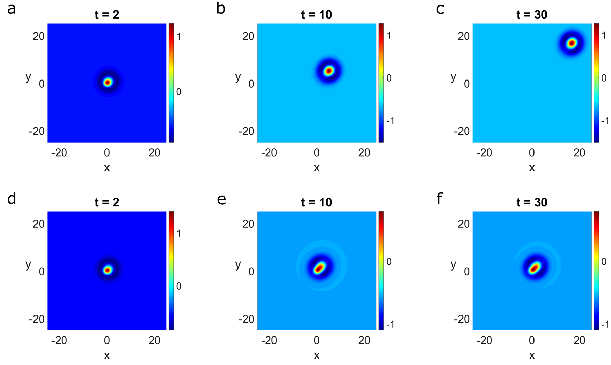
Abstract
Continuous bump attractor networks (CANs) have been widely used in the past to explain the phenomenology of working memory (WM) tasks in which continuous-valued information has to be maintained to guide future behavior. Standard CAN models suffer from two major limitations: the stereotyped shape of the bump attractor does not reflect differences in the representational quality of WM items and the recurrent connections within the network require a biologically unrealistic level of fine tuning. We address both challenges in a two-dimensional (2D) network model formalized by two coupled neural field equations of Amari type. It combines the lateral-inhibition-type connectivity of classical CANs with a locally balanced excitatory and inhibitory feedback loop. We first use a radially symmetric connectivity to analyze the existence, stability and bifurcation structure of 2D bumps representing the conjunctive WM of two input dimensions. To address the quality of WM content, we show in model simulations that the bump amplitude reflects the temporal integration of bottom-up and top-down evidence for a specific combination of input features. This includes the network capacity to transform a stable subthreshold memory trace of a weak input into a high fidelity memory representation by an unspecific cue given retrospectively during WM maintenance. To address the fine-tuning problem, we test numerically different perturbations of the assumed radial symmetry of the connectivity function including random spatial fluctuations in the connection strength. Different to the behavior of standard CAN models, the bump does not drift in representational space but remains stationary at the input position.
Links
DOI PDFBibTeX
@article{wojtakRobust2023,
author = {Wojtak, Weronika and Coombes, Stephen and Avitabile, Daniele and Bicho, Estela and Erlhagen, Wolfram},
title = {Robust working memory in a two-dimensional continuous attractor network},
issn = {1871-4080, 1871-4099},
url = {https://link.springer.com/10.1007/s11571-023-09979-3},
doi = {10.1007/s11571-023-09979-3},
journal = {Cognitive Neurodynamics},
month = may,
year = {2023},
}